Integrating artificial intelligence (AI) into desalination and water reuse plants has become imperative to elevate operational efficacy and reduce energy consumption. Over the last several years, pressure to bid lower and lower values on large reverse osmosis (RO) plants has increased substantially, with some large RO systems at less than USD 0.30/m3. In response to escalating pressures to curtail costs in RO plants, as well as meet carbon footprint and sustainability initiatives provided by governments, the strategic application of machine learning (ML), a subset of AI, has emerged as an indispensable tool for plant management.
One such example of this endeavor has been Gradiant’s digital AI team, Turing, partnering with PUB, Singapore’s National Water Agency. Leveraging our SmartOps Digital AI platform, we’ve strategically identified the optimal schedule for cleaning the systems RO membranes at the Bedok NEWater Factory, one of the first water reuse plants in Singapore. SmartOps Digital is an integrated platform that utilizes machine learning AI algorithms for the optimization and prediction of plant operations. It uses historical and real-time process data to deliver immediate productivity improvements and cost savings, as well as reduce the carbon and water footprints in systems where it’s installed.
Traditionally, energy optimization has been a focal point in seawater RO plants to minimize costs. However, water reuse plants, characterized by lower consumption, necessitate nuanced optimization strategies. The Bedok NEWater Factory, operational for over 20 years, sought to obviate membrane replacements and associated costs by strategically determining the optimal time to clean the RO membranes.
The project, with a primary focus on mitigating irreversible fouling induced by biofouling, engaged SmartOps AI algorithms by our team, in collaboration with PUB Singapore. The algorithm meticulously analyzed daily data, specifically honing in on Normalized Differential Pressure (DP), to discern the onset of biofouling. The algorithm categorized risk conditions – Yellow, Orange, and Red – signifying the opportune time for cleaning. Rigorous testing at Bedok demonstrated an exceptional accuracy rate of 98.1% in predicting cleaning recommendations when the plant is operating under normal conditions. Figure 1 shows real data from the Bedok plant, indicating a Yellow, Orange, and Red risk category.
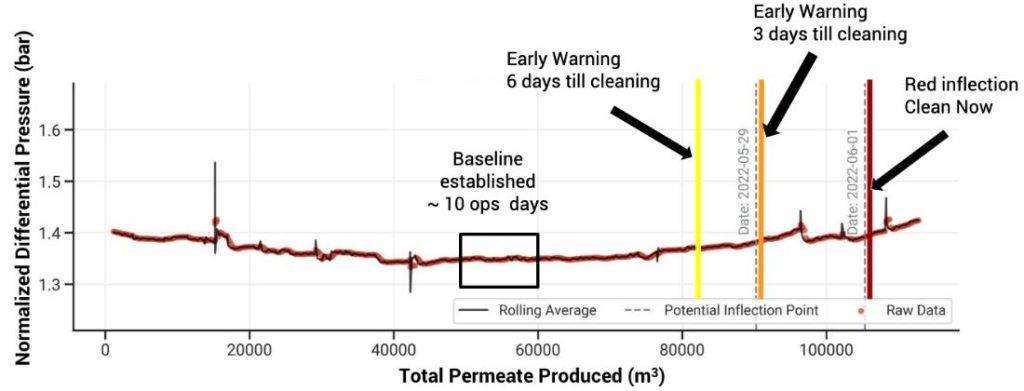
Through predictive analytics, SmartOps optimizes the cleaning schedule as illustrated in the graph. This can potentially decrease the necessity for replacements and contribute to a diminished carbon footprint associated with the manufacturing and transportation of new membranes.
The Bedok NEWater Factory, commissioned in 2002, boasts a daily production capacity of 82,000m3 of recycled water earmarked primarily for industrial applications in Singapore. The trial unfolded in three precise phases: historic data analysis, live recommendations incorporating inputs from the Bedok operations team, and live recommendations devoid of inputs from the Bedok operations team on operational events.
The results were as follows, in Phase 1, the historical data analysis underscored the AI algorithm’s prowess in detecting inflections in Normalized DP, a pivotal precursor for predicting biofouling. The analysis revealed a remarkable accuracy rate of 98.1% in predicting the imperative need for cleaning to avert irreversible fouling. Noteworthy, the five instances of missed inflections were attributed to sporadic data inconsistencies.
Phase 2 involved the delivery of live recommendations to Bedok operators, who keenly observed trends without immediate implementation. The analysis illuminated the discernment that specific operational events, such as permeate flushes and mode switches, could attenuate the necessity for cleaning, thereby informing subsequent refinements to the algorithms.
Phase 3 constituted live recommendations without inputs from Bedok on operational events, constituting a blind test to independently validate the AI algorithm.
In conclusion, the demonstration of AI at the Bedok NEWater Factory stands as a testament to the transformative potential of AI in optimizing water reuse plant operations and making meaningful contributions to carbon capture initiatives. By accurately predicting the opportune time to clean RO membranes, AI not only demonstrates its efficacy in operational cost reduction but also holds promise for substantial extension of membrane lifespans and broader environmental goals. As this project evolves, the industry can anticipate further strides in water treatment plant efficiency and sustainability practices through the discerning application of state-of-the-art AI technologies.
Article by Gradiant Team:
Mike Dixon, First Author
Prakash Govindan, Second
Author Hiep Le, Third Author